Is there a Signal in all that Noise? GWAK’s Search for Unmodeled Gravitational Waves
By: Katrine Kompanets
March 4, 2025
First predicted by Einstein’s theory of General relativity over 100 years ago, gravitational wave detection by the Laser Interferometer Gravitational-Wave Observatory (LIGO) has improved greatly since its first detection in 2015. Now, joined by the Virgo and KAGRA observatories, in the middle of its 4th observing run, LIGO has recorded almost 100 confirmed signals, all of which are ‘modeled’ compact object coalescences (CBCs) from binary black holes and neutron stars. These types of signals are known models since these collisions follow a well-defined waveform template. This template is then used to find the incredibly small disturbance in spacetime captured by the interferometers as the gravitational wave passes through Earth.
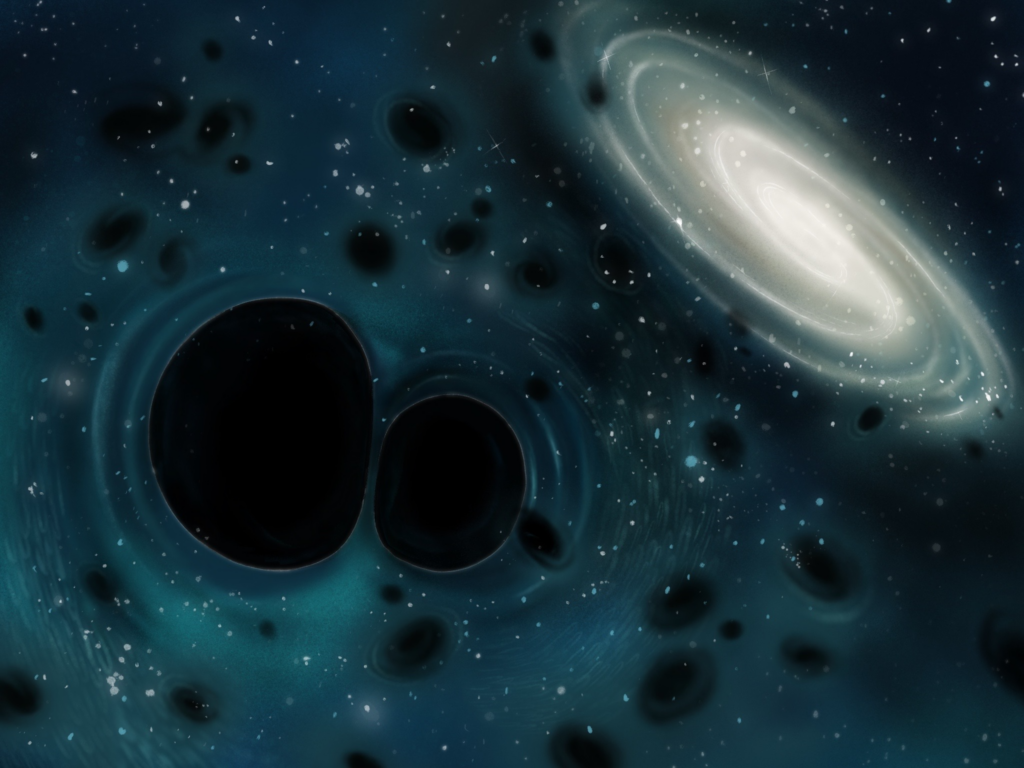
Gravitational Wave Anomalous Knowledge (GWAK) is a neural network-based technique designed for the detection of unmodeled gravitational wave signals. Unmodeled signals, like those from core-collapse supernovae, are different from modeled signals because they don’t have a well-defined waveform. Heavy reliance on template waveforms means these signals can’t be detected the traditional way, which uses a matched filtering technique. Building on the method described here, GWAK uses semi-supervised learning to look for unmodeled signals. By training autoencoders on different types of data like detector noise, glitches, and simulated CBC signals, GWAK creates a low-dimensional representation of data allowing the network to easily distinguish signals from noise.
Testing using the LIGO-Virgo-Kagra collaboration’s complete catalogue of data from its third observing run revealed that while GWAK didn’t identify any statistically significant unmodeled signals, it was able to maintain sensitivity in high-glitch periods where many searches avoid analysis due to intense noise. This robustness across a broad range of noise types, as well as detection of confirmed CBC signals, demonstrate GWAK’s potential to broaden the scope of gravitational wave detection. Its ability to analyze traditionally discarded data opens new possibilities for future discovery. Incorporation of heuristic models to down-weight glitch-like events and frequency-domain correlations to reduce false alarms demonstrate its adaptability in addressing real detection challenges.
Overall, this study lays the foundation for GWAK’s potential in upcoming observing runs, offering an exciting opportunity to explore the universe in a novel way.