HDR Ecosystem
NSF’s Harnessing the Data Revolution (HDR) Big Idea is a national-scale activity founded in 2016 to enable new modes of data-driven discovery that address fundamental questions at the frontiers of science and engineering. By engaging NSF’s research community in the pursuit of fundamental research in data science and engineering, the ecosystem of HDR Institutes, Data Science Corps (DSC), and Transdisciplinary Research in Principles of Data Science (TRIPODS) strive to provide a cohesive, federated, national-scale approach to research data infrastructure, and the development of a 21st-century data-capable workforce.
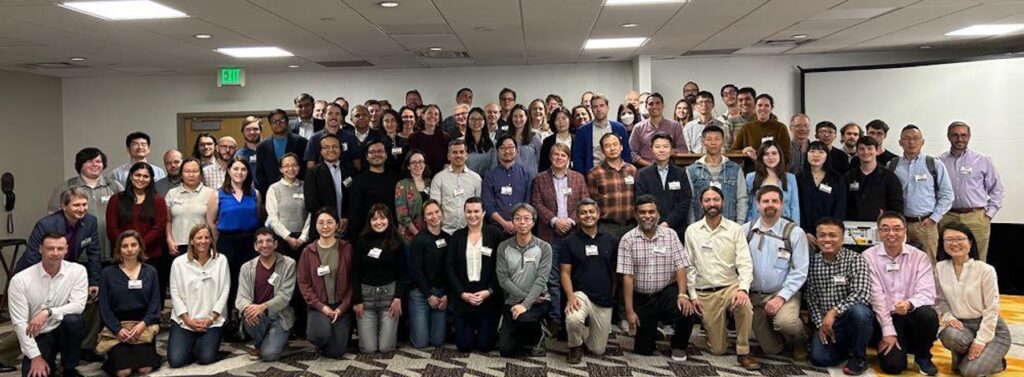
A3D3 is one of five institutes funded by NSF under the Harnessing the Data Revolution (HDR) program. The others are:
- ID4 (Institute for Data Driven Dynamical Design) (https://www.mines.edu/id4/)
- Imageomics (https://imageomics.osu.edu)
- iHARP (Institute for Harnessing Data and Model Revolution in the Polar Regions) (https://iharp.umbc.edu)
- I-GUIDE (Institute for Geospatial Understanding through an Integrative Discovery Environment) (https://iguide.illinois.edu/)
While each institute has its own particular focus, there are a number of initiatives within the HDR ecosystem to build community and to address transdisciplinary issues. Members of all HDR institutes contribute to these activities.
Conferences
An annual conference is held to strengthen relationships among HDR entities, to learn from each other in regard to best practices and innovation, and to develop new collaborations focused on common challenges.
- 2022 NSF HDR Ecosystem Conference, October 26 – 27, Alexandria, VA (https://indico.cern.ch/event/1174814/)
- 2023 NSF HDR Ecosystem Conference, October 16 – 18, Denver, CO (https://www.mines.edu/id4/hdr-conference/)
- 2024 NSF HDR Ecosystem Conference, September 9 – 12, Champaign-Urbana, IL (https://indico.cern.ch/event/1364455/)
Workshops
A number of workshops are being planned within the HDR ecosystem focused on specific issues.
- Integration of Data Fluency into 6th – 12th Grade Education, July 11 – 12, 2024, Golden, CO (URL to follow)
Resources
At the 2023 HDR Ecosystem Conference, a number of common areas of interest between HDR institutes were identified where the development of specific common resources could be beneficial to all HDR entities.
Education Repository (under development)
There is a strong degree of overlap in the education activities of HDR institutes, e.g., the development of general data science courses, and a central repository of curated resources would help the generation of new courses as well as existing ones by providing a wider range of worked examples, problem sets, data sets, etc. to draw on than just those in one’s own scientific domain. Items in the repository would be discoverable based on the type of resource, the type of activity, the education level of the intended audience, and so on.