By: Patrick McCormack (Postdoc MIT, A3D3)
October 30, 2023
At the workshop, seven A3D3 trainees gave presentations on their work. Leading off, Farouk Mokhtar of UCSD and Santosh Parajuli of UIUC presented their work on implementing machine learning models for the LHC experiments CMS and ATLAS, respectively. Though working on independent efforts, both use graph neural networks to efficiently and scalably reconstruct particles. Alongside the first smatterings of autumn leaves, an international assortment of more than 150 Physicists, Computer Scientists, Engineers (and more) descended upon Imperial College London (ICL) this past week. There they enjoyed the crisp weather and the fourth iteration of the Fast Machine Learning for Science (FastML) Workshop, which ran from September 25-28.
The FastML workshop series was born in 2019 as a small and informal workshop focused on High Energy Physics (HEP), but it has since grown to include participants from diverse fields, such as medicine, astrophysics, and statistics. Unsurprisingly, a workshop centered on this multidisciplinary approach to accelerated machine learning drew the participation of several members of A3D3. And just as A3D3 revolves around mutual support and cross-disciplinary efforts, participants in the workshop were intrigued to see how the same techniques and algorithms were found in diverse applications across different disciplines.
“The workshop brings together researchers from very different specialties who do not typically have a chance to come together and exchange ideas,” said Fermilab’s Nhan Tran, one of the original FastML organizers. “Despite this, it was so refreshing to see many amazing talks and enthusiastic discussion from all the workshop participants willing to get out of their comfort zones and expand their research. I really appreciate that spirit and it makes the workshop series very unique and fun.”
Emphasizing the increased scope of the workshop series, Fermilab’s Kevin Pedro said that he attended the workshop “to learn about new cutting-edge computational techniques that are accelerating ML throughout many scientific fields.”
At the workshop, seven A3D3 trainees gave presentations on their work. Leading off, Farouk Mokhtar of UCSD and Santosh Parajuli of UIUC presented their work on implementing machine learning models for the LHC experiments CMS and ATLAS, respectively. Though working on independent efforts, both use graph neural networks to efficiently and scalably reconstruct particles.
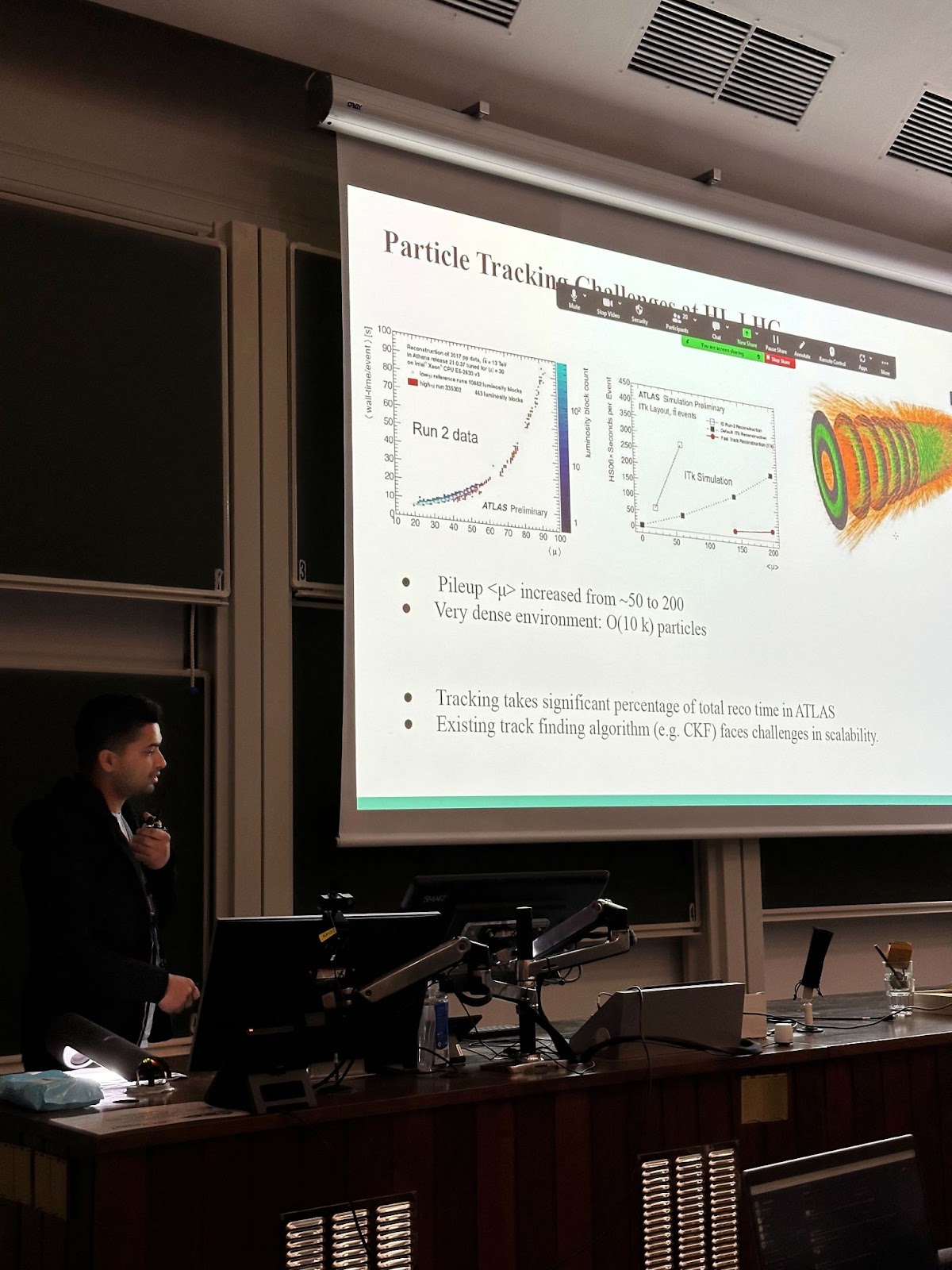
Jeffrey Krupa presents his work on developing a Sparse Point Voxel CNNSantosh Parajuli presents his work on implementing graph neural networks for Event Filter Tracking in ATLAS. In keeping with the “Fast” theme of the workshop, he is developing a VHDL implementation of his algorithm to run on FPGAs. for machine-learning-based clustering in hadronic calorimeters.
Next up, Patrick McCormack and Jeffrey Krupa, both of MIT, gave talks about two projects that they both work on. One is a CMS effort to implement GPU acceleration for ML-methods via an Inference as a Service scheme, and the other is an implementation of a Sparse Point Voxel CNN (SPVCNN) for determining clusters of energy in hadron calorimeters for LHC experiments. According to Krupa, “the SPVCNN algorithm is a first-time use of HCAL depth segmentation in clustering for CMS, and it removes the latency associated with HCAL clustering from reconstruction workflows.”
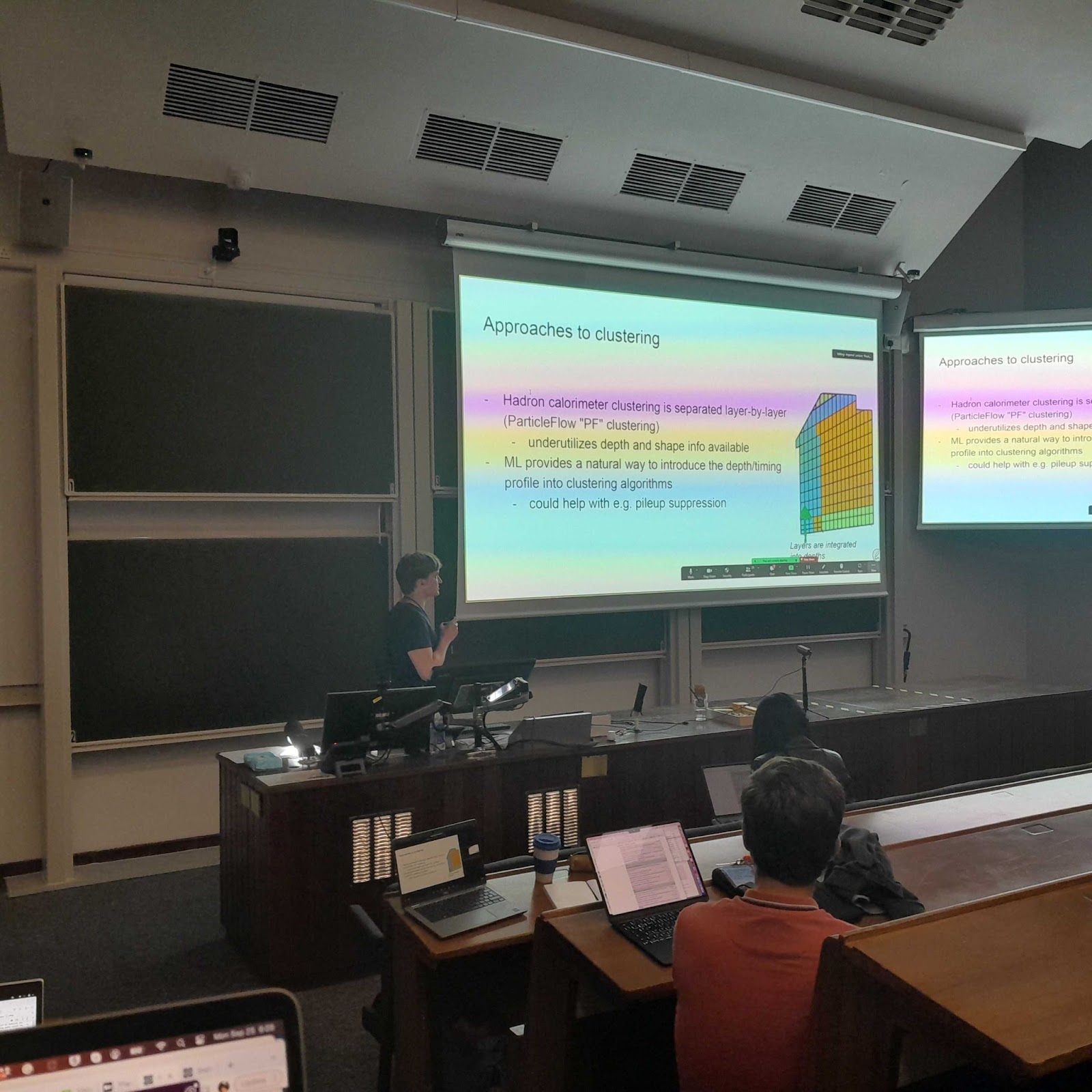
Jeffrey Krupa presents his work on developing a Sparse Point Voxel CNN for machine-learning-based clustering in hadronic calorimeters.
The last A3D3 talk from the workshop’s first day was given by Duc Hoang, also from MIT, who presented his work on algorithms for the CMS Layer-1 Trigger. These algorithms must be able to produce inferences at 40 MHz, such that one must balance algorithmic complexity with speed. Thanks to the efforts of Duc and his collaborators, the algorithms that they have implemented on FPGAs for both bottom quark and tau lepton identification will increase the efficiency of the CMS trigger system for rare processes with these particles.
During the second day of the workshop, the focus shifted away from the LHC. The gravitational waves side of A3D3 was represented by Katya Govorkova and Eric Moreno of MIT. Katya presented her work on the development of Gravitational Wave Anomalous Knowledge (GWAK), a method for tagging gravitational waves from anomalous sources. This algorithm is related to the Quasi-Anomalous Knowledge (QUAK) technique that was developed by A3D3 members for application in LHC contexts. The GWAK algorithm has since evolved and can be used in real time to help distinguish between truly anomalous gravitational waves and meaningless detector glitches.
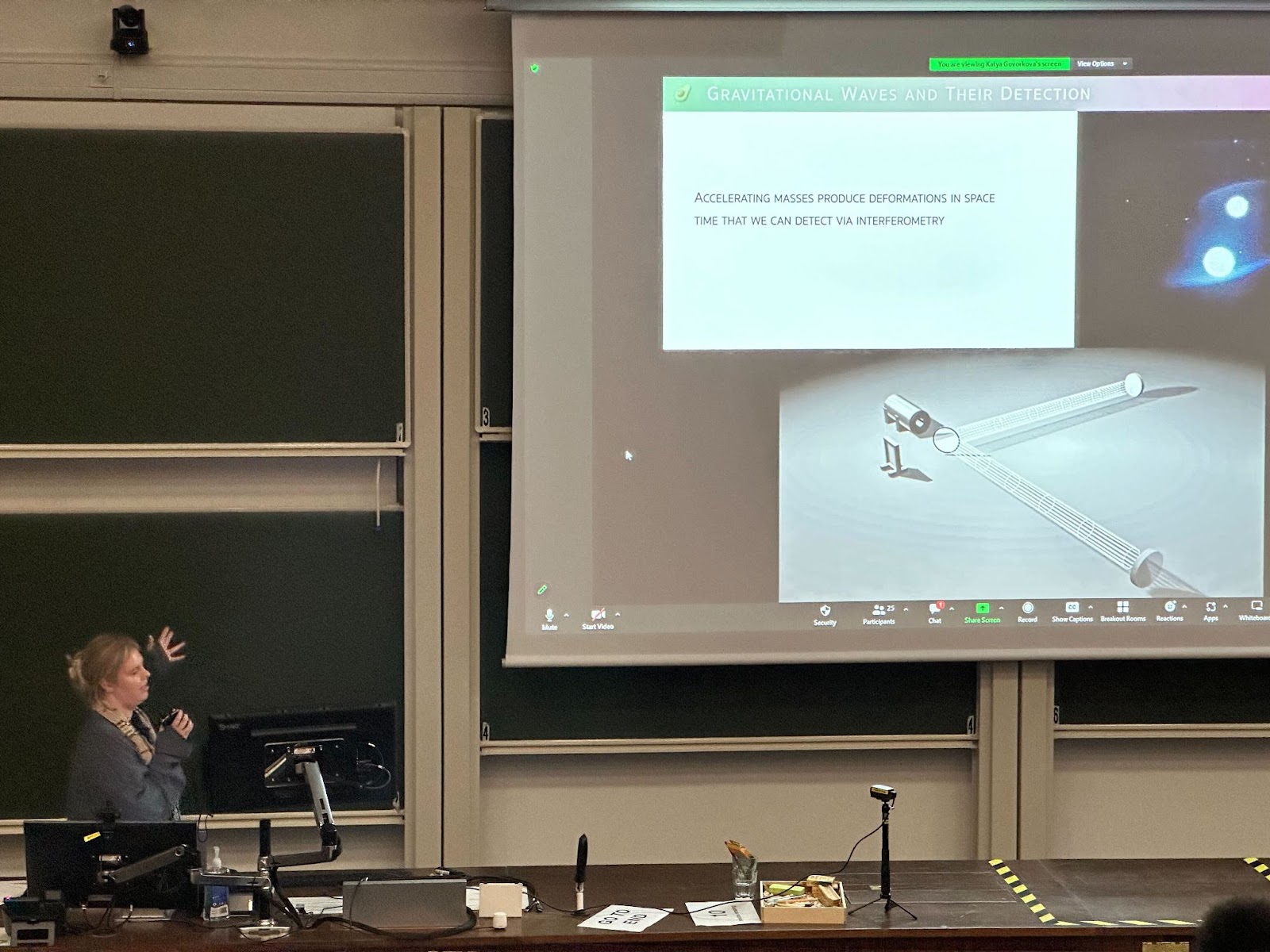
Katya Govorkova presents “Gravitation Wave Anomalous Knowledge”, or GWAK. As she was giving the first talk about gravitational waves at the workshop, she covered the basic physics behind the LIGO experiment.
Eric’s talk focused on the Machine Learning for Gravitational Waves (ML4GW) package, which is a suite of tools enabling real-time machine learning for gravitational wave (GW) experiments, such as LIGO. These tools have accelerated GW-detection, parameter estimation for events, noise regression, and anomaly detection via GWAK.
The workshop’s concluding remarks were given by A3D3’s deputy director and MIT professor Phil Harris. He exhorted the audience with this playful paradox: “In order to go fast, we have to go slow. By which I mean that designing an algorithm or workflow for the fastest possible performance takes time and careful consideration.” In his 20 minute talk, he pointed out many of the similarities and common tools being used across disciplines, such as sparsification and quantization of neural networks, hardware-based acceleration using GPUs and FPGAs, and deep learning architectures such as transformers and graph neural networks. A complete summary of the topics covered in the workshop would be far too long for this article, but the workshop’s timetable, along with links to most presentations can be found here.
“I really enjoyed that the workshop has a very diverse group of participants and talks that I found very inspiring,” said Professor Mia Liu from Purdue reflecting on the workshop. “I am learning and thinking of new ways of accelerating science by developing appropriate algorithms and learning methods, in addition to my current research in real-time ML in low latency and high throughput systems. For example, robust learning methods for embedding of scientific data, that can account for the variance due to the nature of the physical object and the measurement methods etc, is challenging but crucial for broader and long lasting impact of ML on scientific discoveries.”
The workshop also included tutorials on state-of-the-art deployment techniques, such as the hls4ml package for creating firmware implementations of machine learning algorithms, Intel AI Suite for deploying algorithms on Intel FPGAs, and the use of Intelligence Processing Units (IPUs) from Graphcore. There were also informal tours of several of the labs at ICL.
On a lighter note, some workshop participants found time to explore the sights and sounds of London. A3D3 members Eric and Duc also put together a public lecture from rapper Lupe Fiasco (Wasalu Jaco), who is currently a visiting professor at MIT. He discussed some of his work with Google on creating TextFX, which is a large language model for exploring relationships between words and generating phonetically, syntactically, or semantically linked phrases. They also managed to bring in Irving Finkel of the British Museum, who discussed the history of the game of Ur, which is a shared love with Lupe, Eric, and Duc.
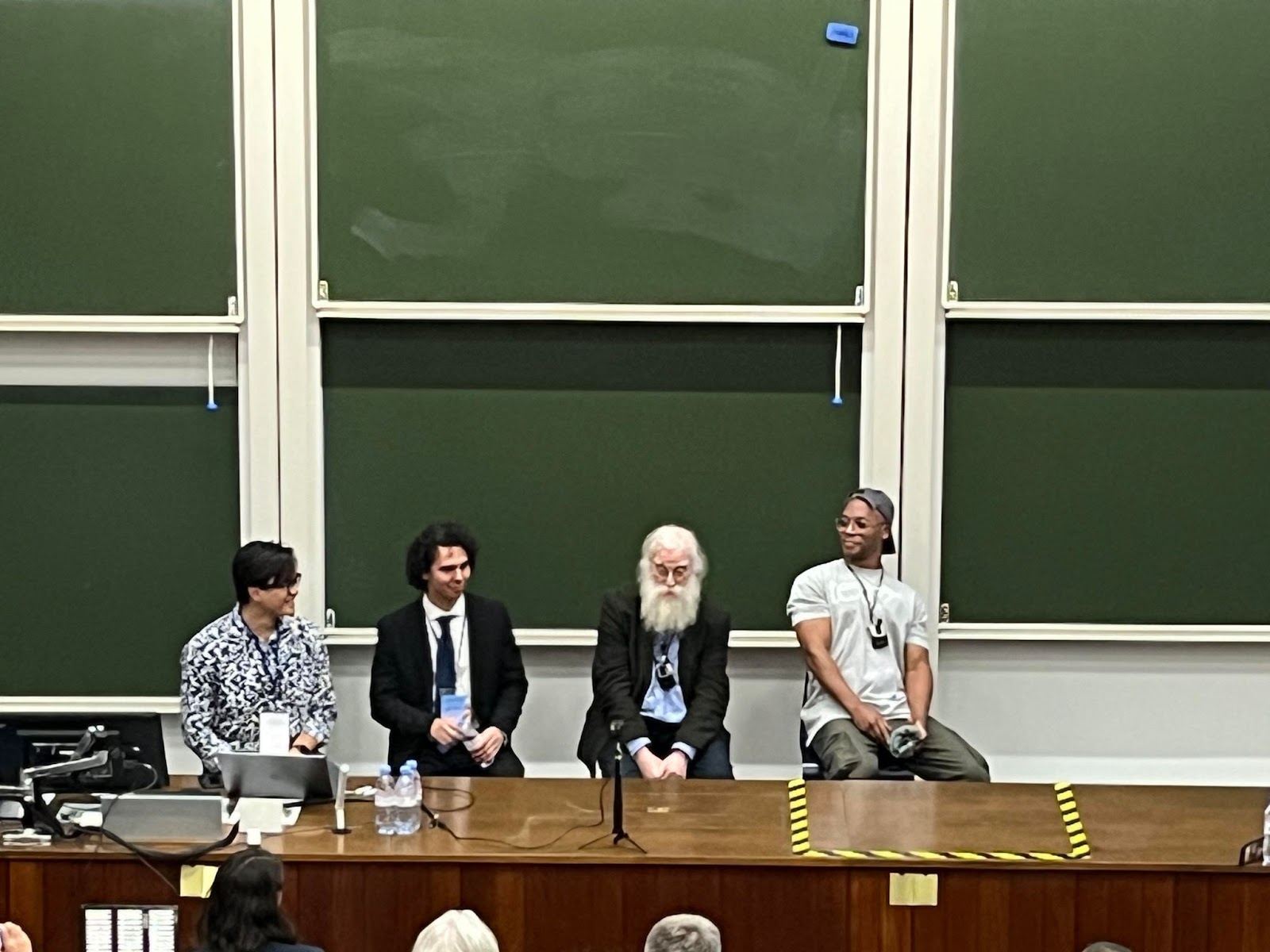
Duc, Eric, Irving Finkel, and Lupe Fiasco discussed the game of Ur in a panel after Lupe’s public lecture on the relationship between rap and large language models.
The workshop proved to be a valuable experience for A3D3 attendees, and I suspect that future iterations will be well attended by our members. “Attending the workshop was an incredible experience for me,” said Santosh Parajuli. “The FastML workshop provided a unique platform to learn from experts, exchange ideas, and explore the latest advancements in different fields. Additionally, I had a chance to share our exciting work on using advanced technology and machine learning to improve how we track particles in high-energy physics, which could help us make big discoveries in the future!”