A3D3 Highlights AI for Scientific Discovery at NeurIPS
By: Eli Chien
December 19, 2024
Vancouver, Canada – The Thirty-Eighth Annual Conference on Neural Information Processing Systems (NeurIPS 2024) was held in Vancouver from December 10 to 15, 2024. NeurIPS is one of the premier machine learning and AI conferences, gathering outstanding researchers from around the world. A3D3 members contributed seven posters, including one spotlight poster and one benchmark poster, and three workshop papers. In addition, A3D3 member Dr. Thea Klaeboe Aarrestad from ETH Zürich was an invited speaker at the WiML (Women in Machine Learning) workshop and the ML4PS (Machine Learning and the Physical Sciences) workshop, presenting “Pushing the limits of real-time ML: Nanosecond inference for Physics Discovery at the Large Hadron Collider.” We are excited to see that A3D3 members are an important part of the machine learning community!
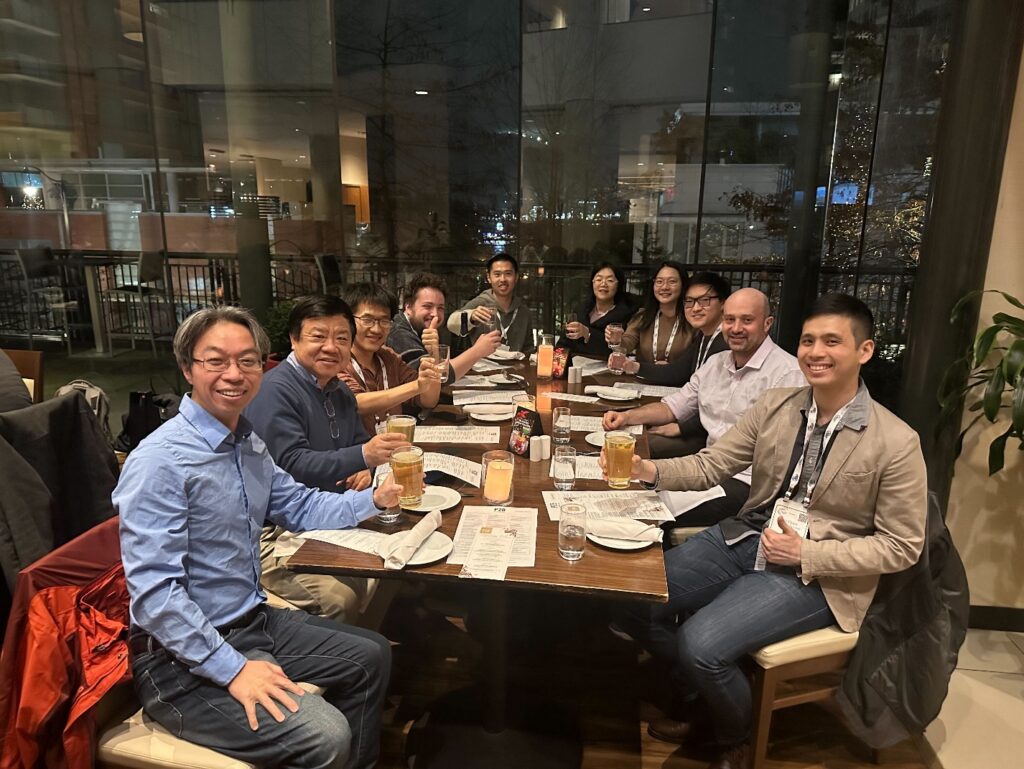
Invited Talks
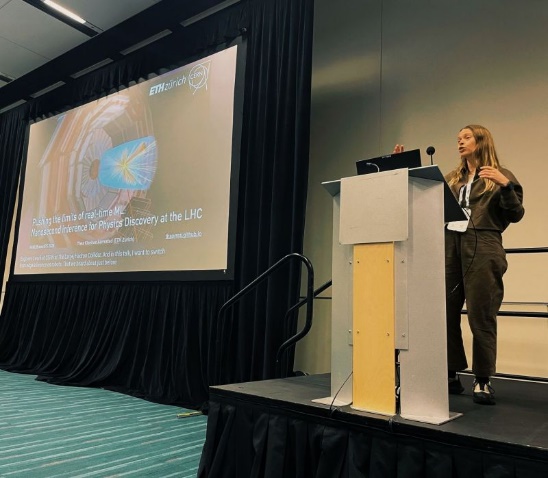
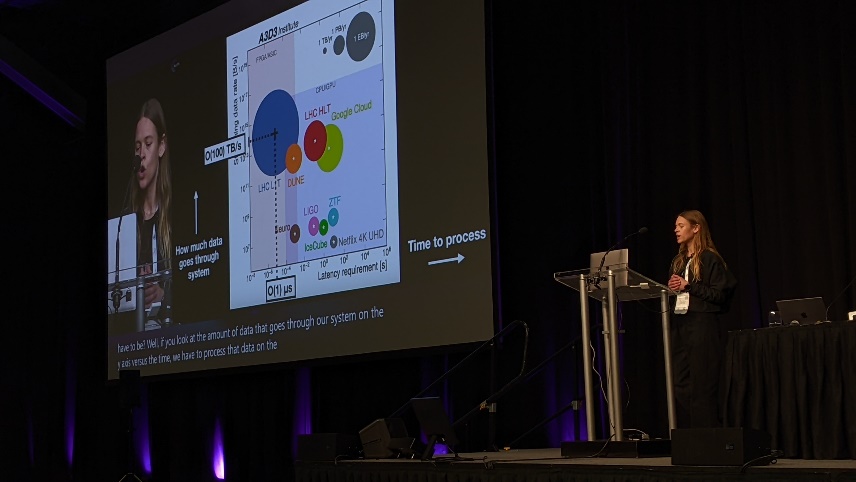
Dr. Thea Klaeboe Aarrestad gives invited talks for the WiML workshop (left) and the ML4PS workshop (right).
Poster Presentations
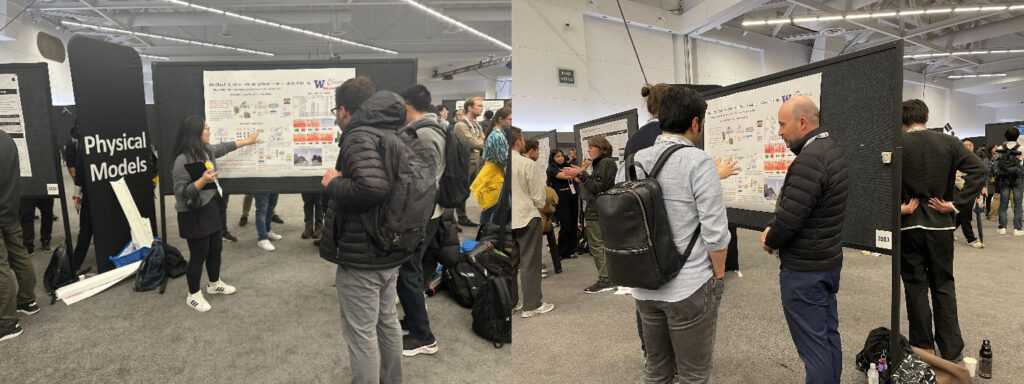
Mingfei Chen (left) and Dr. Eli Shlizerman (right) from the University of Washington presented “AV-Cloud: Spatial Audio Rendering Through Audio-Visual Cloud Splatting.” They propose a novel approach, AV-Cloud, for rendering high-quality spatial audio in 3D scenes that is in synchrony with the visual stream but does not rely on nor is explicitly conditioned on the visual rendering. [paper link, project link]
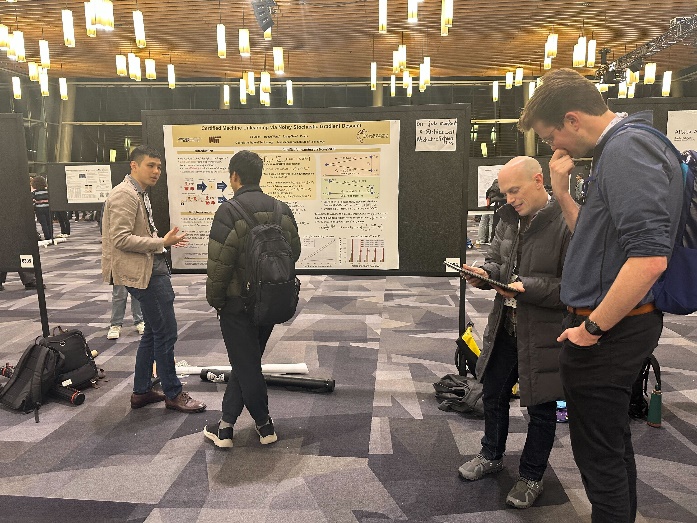
Dr. Eli Chien from the Georgia Institute of Technology presented a spotlight poster titled “Langevin Unlearning: A New Perspective of Noisy Gradient Descent for Machine Unlearning.” They propose Langevin unlearning which not only connects DP with unlearning, but also provides unlearning privacy guarantees for PNGD with Langevin dynamic analysis. [paper link]
Eli Chien also presented a poster titled “Certified Machine Unlearning via Noisy Stochastic Gradient Descent.” They propose to leverage stochastic noisy gradient descent for unlearning and establish its first approximate unlearning guarantee under the convexity assumption. [paper link]
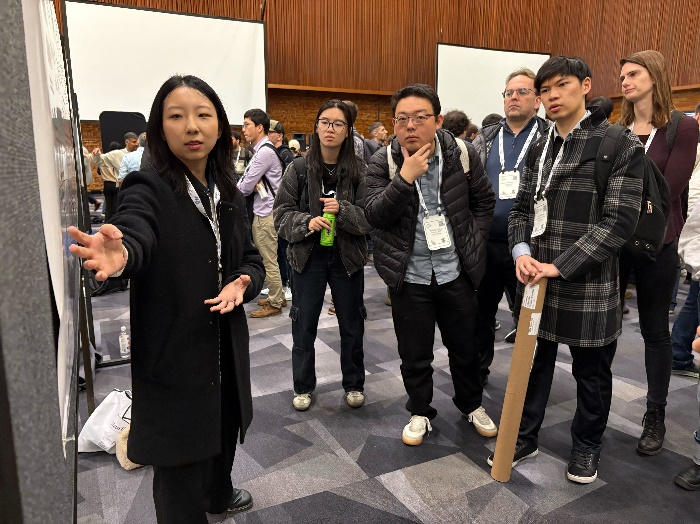
Jingru (Jessica) Jia from the University of Illinois at Urbana-Champaign presented “Decision-Making Behavior Evaluation Framework for LLMs under Uncertain Context.” This paper quantitatively assesses the decision-making behaviors of large language models (LLMs), demonstrating how these models mirror human risk behaviors and exhibit significant variations when embedded with socio-demographic characteristics. [paper link]
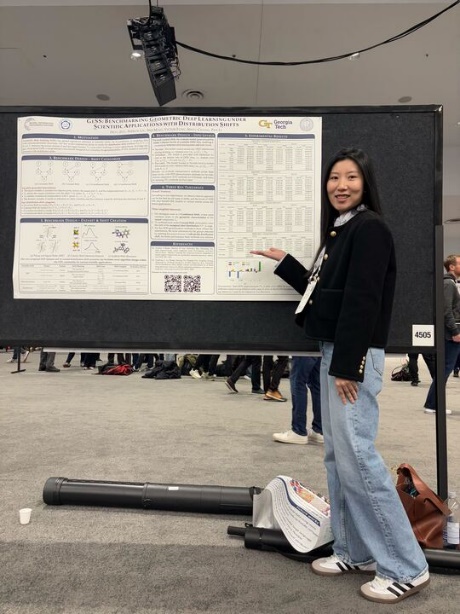
Shikun Liu from the Georgia Institute of Technology presented “GeSS: Benchmarking Geometric Deep Learning under Scientific Applications with Distribution Shifts.” They propose GeSS, a comprehensive benchmark designed for evaluating the performance of geometric deep learning (GDL) models in scientific scenarios with various distribution shifts, spanning scientific domains from particle physics and materials science to biochemistry. [paper link]
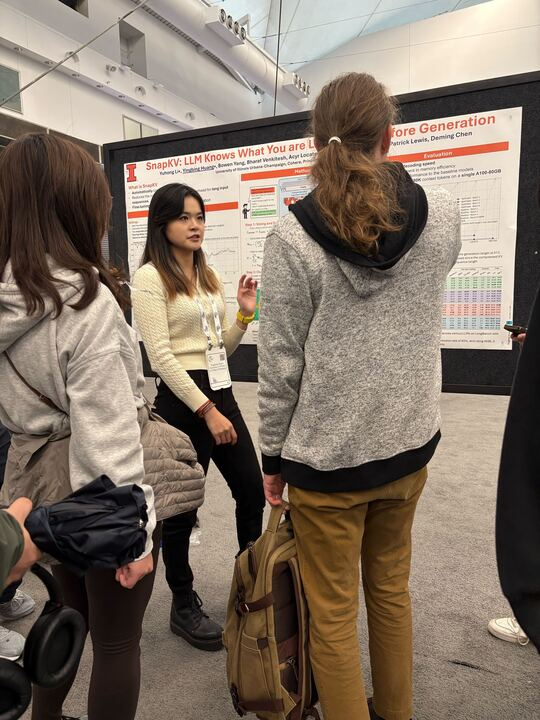
Yingbing Huang from the University of Illinois Urbana-Champaign presented “SnapKV: LLM Knows What You are Looking for Before Generation.” They propose SnapKV, a fine-tuning-free method to reduce the Key-Value (KV) cache size in Large Language Models (LLMs) by clustering important positions for each attention head. [paper link]
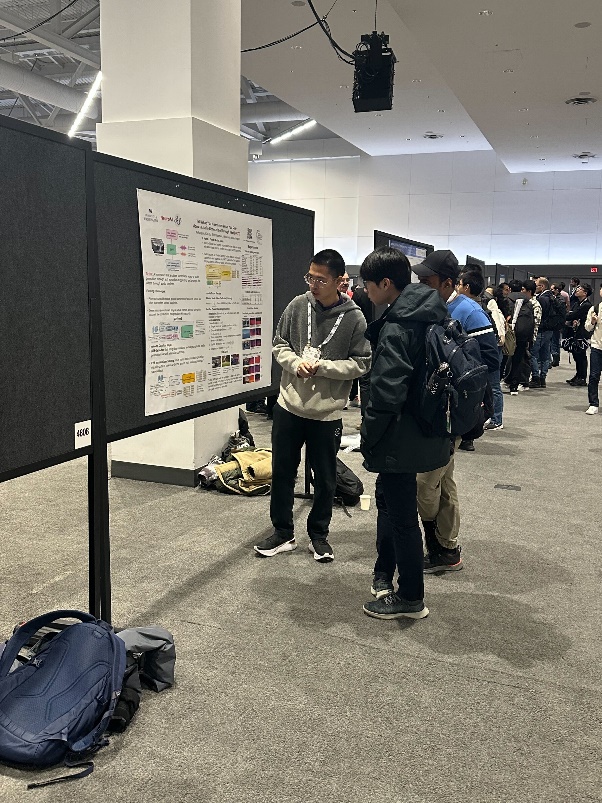
Xiulong Liu from the University of Washington presented “Tell What You Hear From What You See – Video to Audio Generation Through Text.” It is a novel multi-modal generation framework for text guided video-to-audio generation and video-to-audio captioning. [paper link]
Workshop Presentations
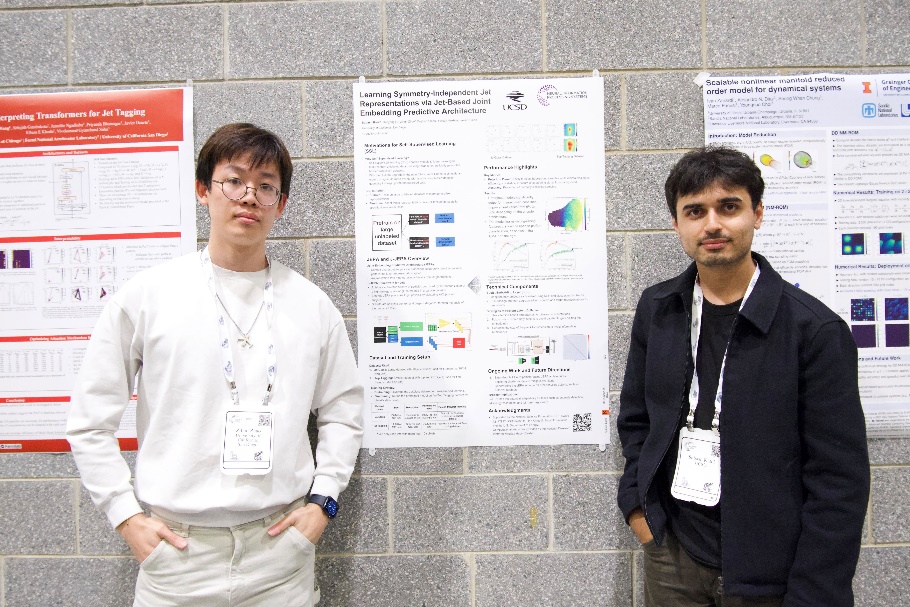
Zihan Zhao from the University of California, San Diego presented “Learning Symmetry-Independent Jet Representations via Jet-Based Joint Embedding Predictive Architecture” at ML4PS workshop. They propose an approach to learning augmentation-independent jet representations using a jet-based joint embedding predictive architecture (J-JEPA). [paper link, project link]
Trung Le from the University of Washington presented “NetFormer: An interpretable model for recovering identity and structure in neural population dynamics” at the NeuroAI workshop. They introduce NetFormer, an interpretable dynamical model to capture complicated neuronal population dynamics and recover nonstationary structures. [paper link]
Andrew “AJ” Wildridge from Purdue University presented “Bumblebee: Foundation Model for Particle Physics Discovery” at ML4PS workshop. Bumblebee is a transformer-based model for particle physics that improves top quark reconstruction by 10-20% and effectively generalizes to downstream tasks of classifying undiscovered particles with excellent performance. [paper link, project link]
Patrick Odagiu from ETH Zurich presented “Knowledge Distillation for Teaching Symmetry Invariances” at SciforDL Workshop. They show that knowledge distillation is just as good as data augmentation for learning a specific symmetry invariance in your data set. [paper link]